Artificial intelligence is now one of the most innovative developments in the IT industry. For this reason, many investors and companies have adopted it. If you’re curious about it, we have something for you!
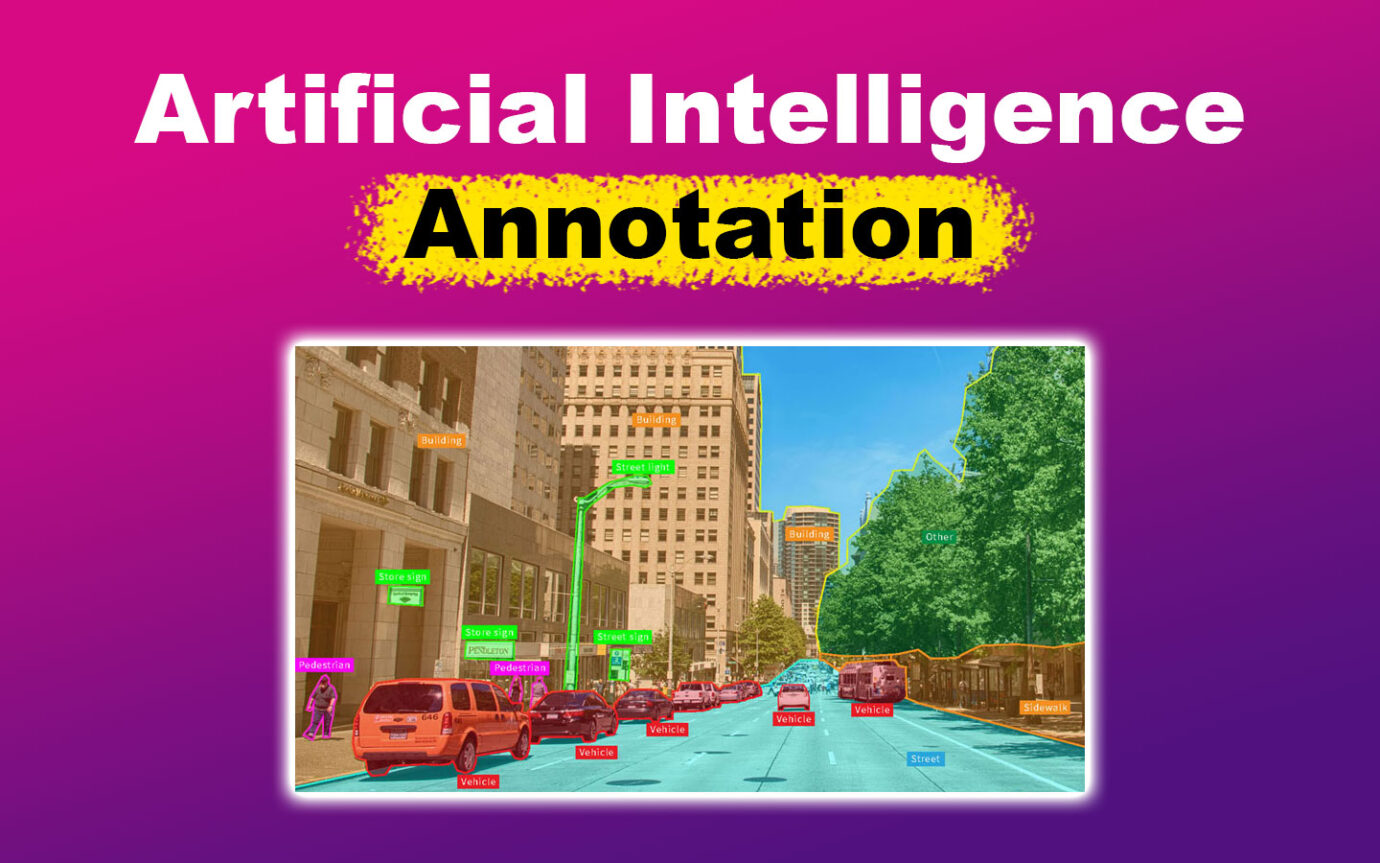
Before diving into the vast world of AI, you should learn some of its basic processes. One of the processes that aid in improving machine learning and artificial intelligence is data annotation.
In this article, we’ll discuss what artificial intelligence annotation means and other details that will help you familiarize yourself with how it works.
What Is Artificial Intelligence Annotation?
Artificial intelligence annotation (AI Annotation) is the practice of labeling or tagging data to enhance its comprehensibility and applicability for machine learning models.
Annotated data plays a pivotal role in training algorithms by furnishing essential input and output pairs that empower the model to recognize patterns and formulate predictions.
According to LinkedIn, different methods of annotating data can be employed and tailored to the particular requirements of an AI model. The annotation process entails the addition of metadata or labels to diverse data types, including images, text, audio, or video.
This augmentation aids the AI system in grasping and identifying specific features or patterns within the data.
The annotated data acts as a training set for supervised learning, enabling the model to learn the mapping of input data to the correct output based on the provided annotations.
In simple terms, artificial intelligence annotation is a bit like giving a computer a helping hand to understand stuff. It’s like adding sticky notes or labels to pictures, text, or sounds so the computer can determine what’s what.
That way, the computer learns from these examples and becomes smarter in recognizing things or making cool predictions.
Here’s a video that explains AI data annotation:
Learn more about the job of a data annotation specialist in this article.
What Is an Example of Data Annotation?
An example of data annotation is categorizing images for self-driving cars. Self-driving vehicles use sophisticated computer vision systems to identify and understand objects in their environment, allowing them to navigate safely and make smart choices.
Data annotation is crucial in the training process of these computer vision models.
Want more examples?
Here are other use cases of data annotation:
- Data Annotation in the Industrial Sector.
Industrial data annotation involves labeling data from manufacturing images, maintenance, safety, and quality control. This annotation is crucial for creating models that detect anomalies in production processes and ensure worker safety. - Data Annotation in the Finance Sector.
This focuses on annotating financial and transactional data. Finance data annotation is critical for developing AI/ML (Artificial Intelligence/Machine Learning) systems that can detect fraud, address compliance issues, and streamline various financial processes. - Data Annotation in the Healthcare Sector.
In healthcare, data annotation involves labeling medical images like MRI scans, electronic medical records (EMRs), and clinical notes. This is essential for constructing computer vision systems for disease diagnosis and automated medical data analysis.
Some companies use dataset annotation tools to annotate production-grade training data for machine learning and AI. Meanwhile, certain organizations opt for a DIY strategy in creating their tools. Numerous data annotation tools are accessible through open-source or freeware options.
Another important form of artificial intelligence is human-in-the-loop machine learning.
What Are the Types of Data Annotation in AI?
These are the types of data annotation in AI:
1. Text Annotation
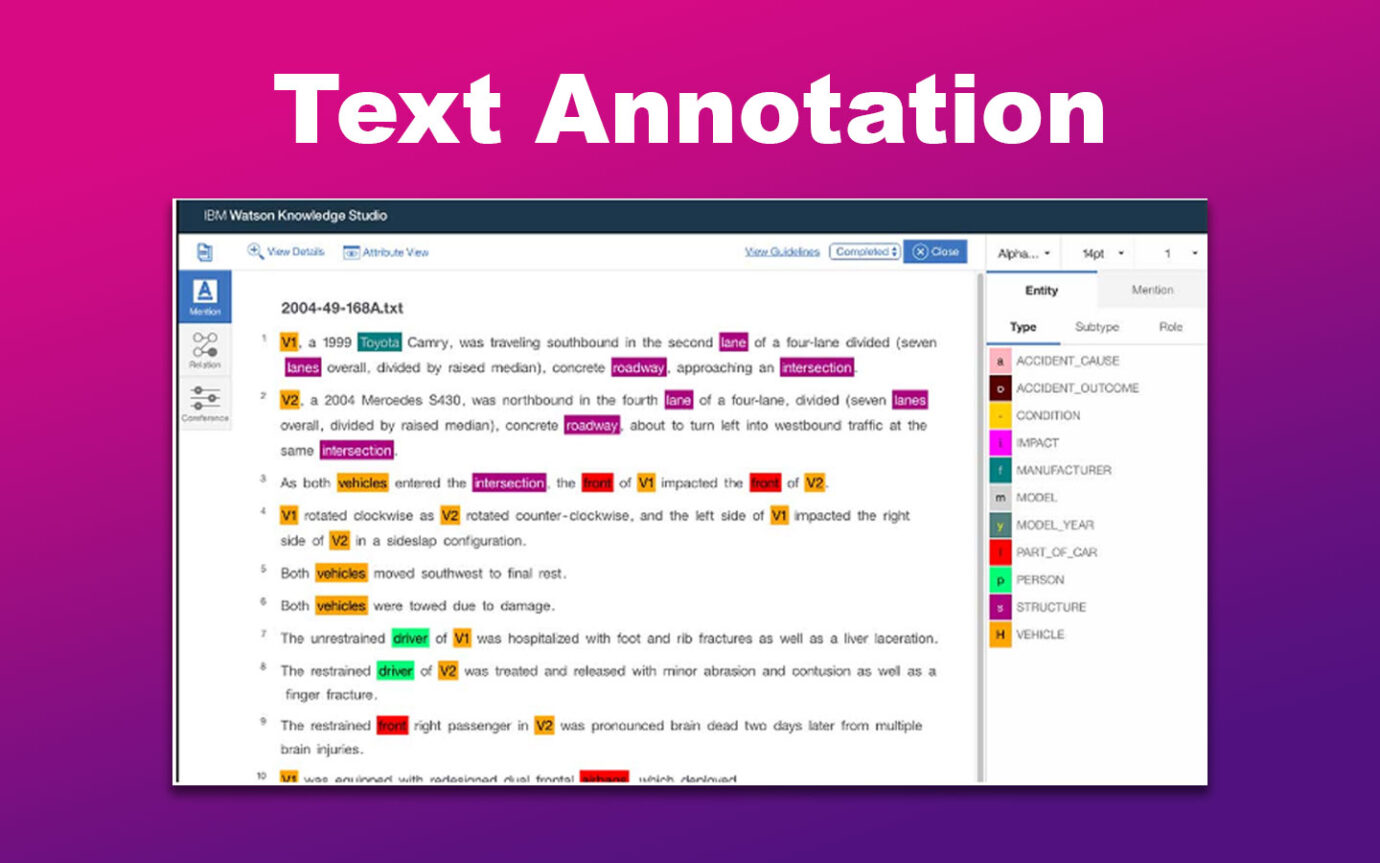
In today’s business world, a lot of important information comes from text-based data, like customer feedback or social media mentions. Unlike images and videos, which are usually straightforward, text has a lot of nuances.
As humans, we naturally grasp the context of phrases, understand word meanings, and connect them to situations or conversations to get the overall meaning. However, machines need help with this precision.
Concepts like sarcasm and humor are unfamiliar to them, making text data labeling challenging. That’s why artificial intelligence text annotation has specific stages.
These are the specific stages of text annotation:
- Semantic Annotation.
This involves making objects, products, and services more relevant through keyphrase tagging. It helps chatbots mimic human conversations. - Intent Annotation.
Tagging the user’s intention and language helps machines understand whether it’s a request, command, recommendation, or something else. - Sentiment Annotation.
This labels text with sentiments like positive, negative, or neutral. It’s often used in sentiment analysis, where AI models learn to understand and evaluate the emotions expressed in text.
2. Image Annotation
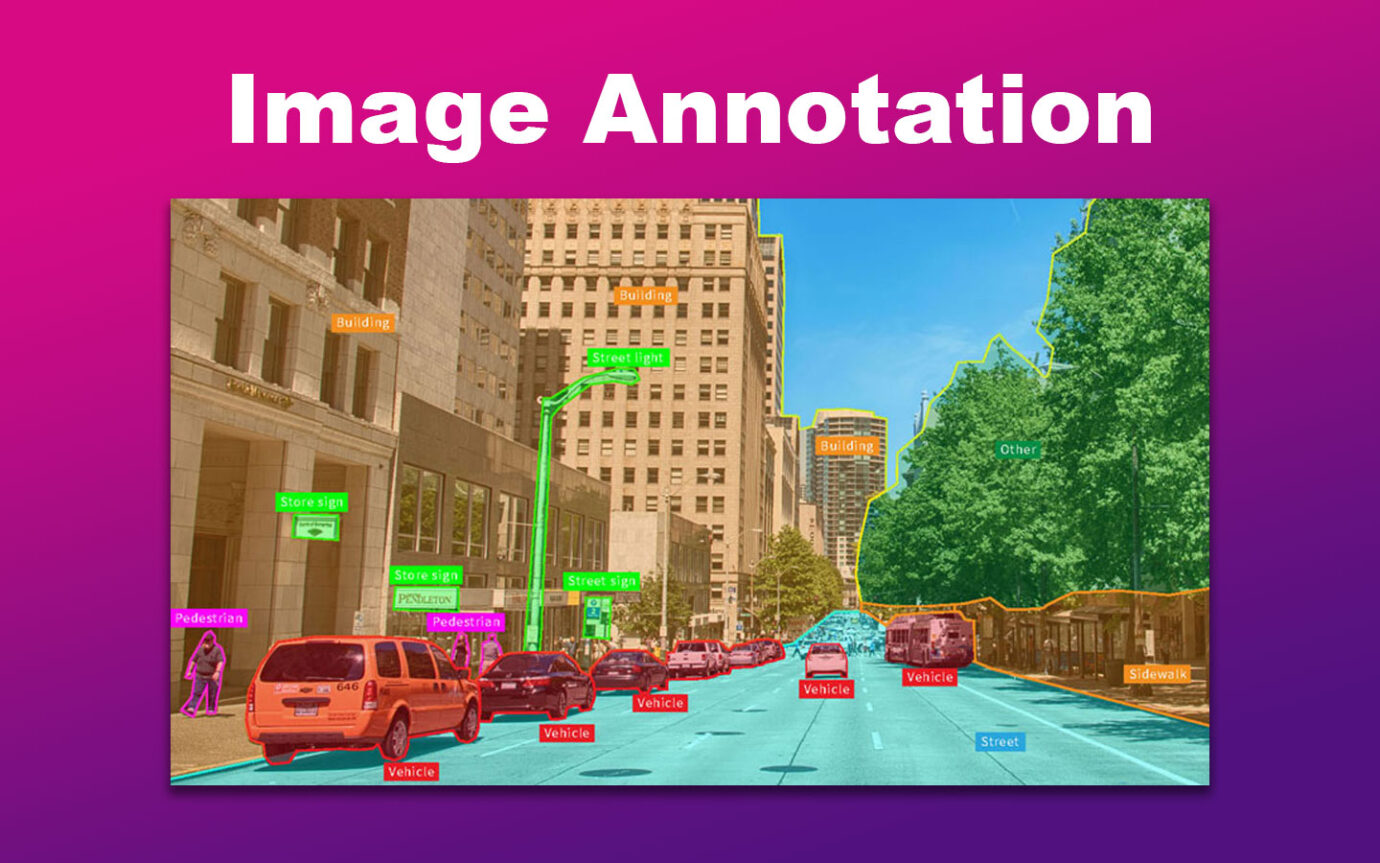
Image annotation involves labeling images to train AI or ML models, enhancing their comprehension, similar to humans when interpreting visual data. Data annotation assigns labels to objects within images, and the number of labels may vary based on the use case.
These are the key types of image annotation:
- Image Classification.
Trained with annotated images, a machine determines what an image represents based on predefined annotations. - Object Recognition/Detection.
A more detailed version of image classification precisely describes the number and positions of entities in the image, labeling entities individually. - Segmentation.
An advanced form of image annotation, segmentation divides the image into image objects for easier analysis. It includes semantic segmentation, instance segmentation, and panoptic segmentation.
Semantic segmentation labels similar objects based on properties like size and location. Instance segmentation individually labels each entity with properties such as position and quantity. Meanwhile, panoptic segmentation combines both approaches for a comprehensive understanding of images.
3. Audio Annotation
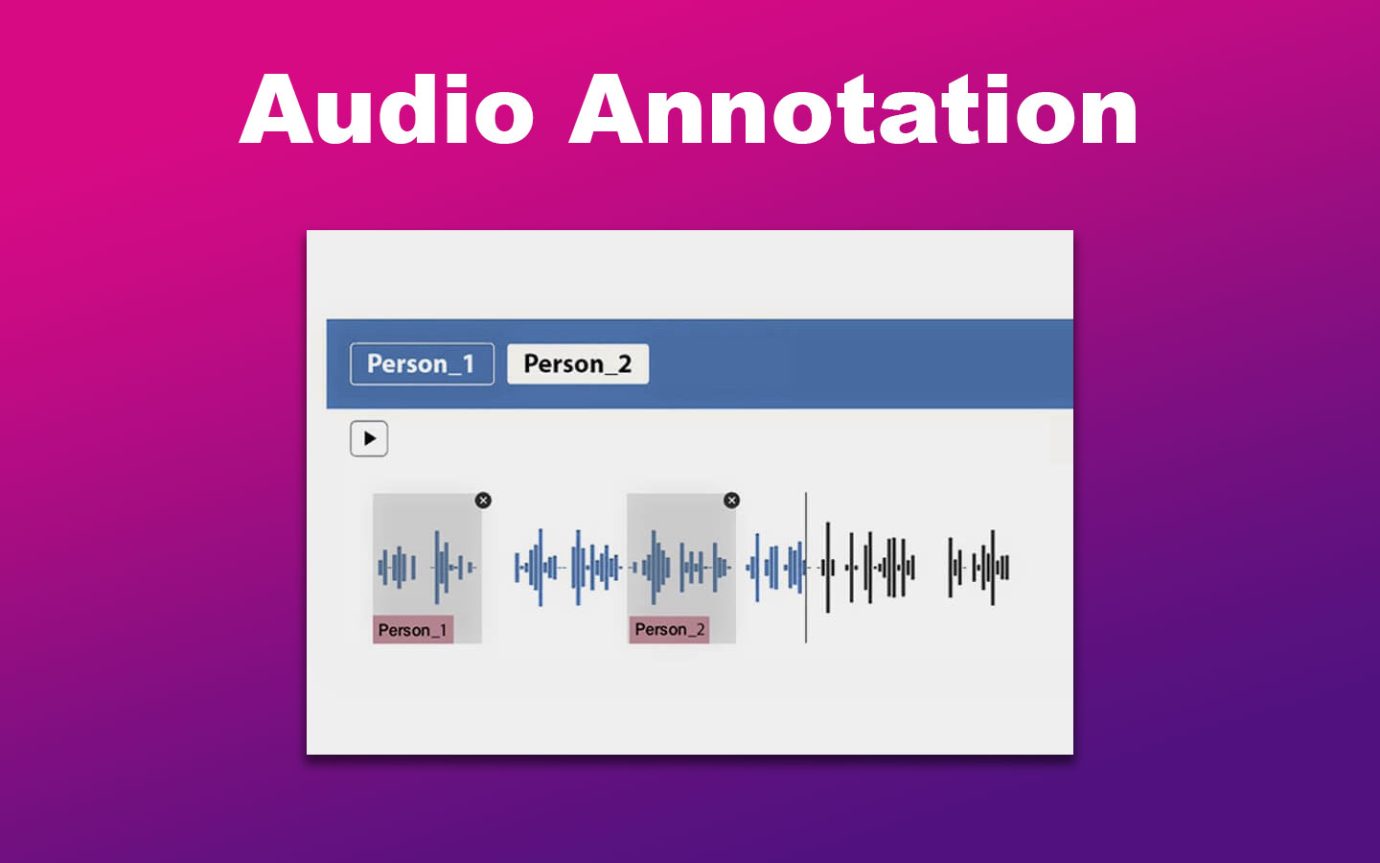
Audio data is like a treasure trove of information—it’s not just about what’s being said! Imagine all the cool things hidden in an audio file, like language, who’s talking, the vibe, feelings, and even background sounds.
To help computers get it, annotators use tricks like putting timestamps, labeling, and more. It’s not just about the words; it’s also about the pauses, breaths, and background noises—all these little details help computers get what’s going on.
4. Video Annotation
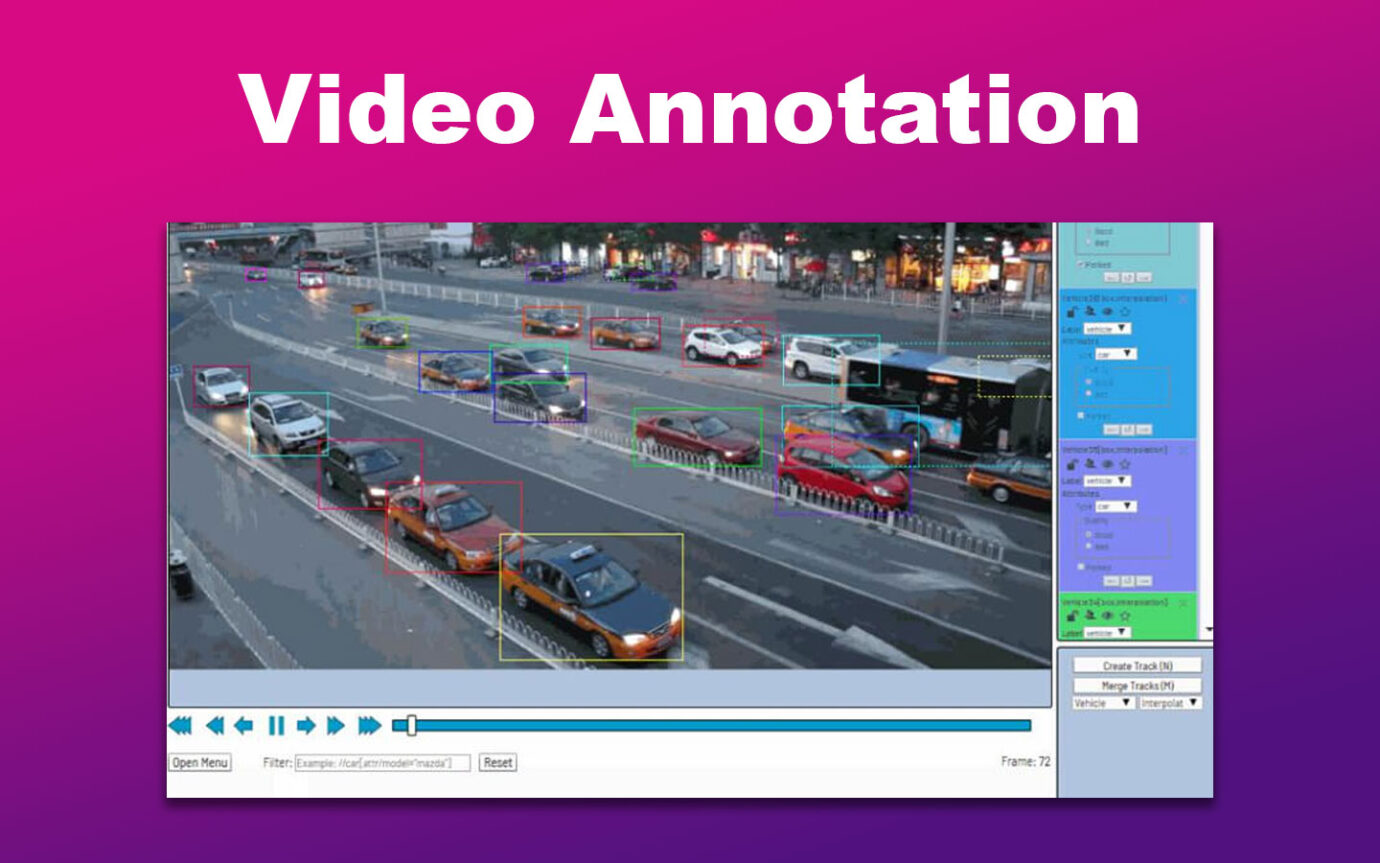
Video annotation in artificial intelligence involves instructing computers to identify objects within videos. Both image and video annotation are methods of annotating data used to train computer vision (CV) systems, which fall under the umbrella of artificial intelligence.
Imagine video annotation like giving eyes to machines. The main job is to spot the relevant stuff in each frame, making it easy for machines to recognize things.
So, for self-driving cars, it’s like teaching them to see pedestrians, street lights, signs, traffic lanes, and all the action on the road.
To become a data annotator, you must first take a data annotation starter assessment to ensure that you have sufficient knowledge about artificial intelligence and machine learning.
What Is the Impact of Data Annotation?
The impact of data annotation extends to machine learning models’ precision, dependability, and effectiveness. This helps AI systems become smarter and more accurate in tasks like recognizing objects in pictures or understanding sentiments in text.
Data annotation is the key to making computers learn and perform various tasks effectively.
Related Post: 5 Advantages of Using AI in the Workplace
Challenges of Artificial Intelligence and Data Annotation
Here are the challenges of artificial intelligence and data annotation:
- Creating Good Annotations Quickly.
Many businesses struggle with production speed, especially when relying only on human annotators for complex tasks. This can slow down your data supply chain and project delivery. - Ensuring Consistent Annotations.
Consistency is crucial for maintaining good data quality during annotation. Low data quality can mess up the results and impact the accuracy of your machine-learning model. - Subjective Labeling.
Different annotators may interpret and label data differently based on personal judgments. This variability introduces inconsistencies in labeled datasets, impacting machine learning model performance.
Clear guidelines and communication are essential to address subjective differences and ensure annotation consistency. -
Tightening Security.
Data safety is crucial for tech professionals, including data annotators. While it’s clear not to share personal or identifying information, some organizations might neglect to take extra precautions to ensure their data is secure. - Not Enough Manpower for Large Data.
A big challenge for organizations is having enough data to train AI models. With insufficient data, progress can be really slow. Annotation, which requires patience and expertise, is tough when there aren’t enough resources to handle large-scale labeling.
Check out this LinkedIn article to learn more about the challenges of data annotation in AI and how to overcome them.
Is There Any Future in Data Annotation?
Yes, there’s a future in data annotation. It’s crucial for training machine learning models and advancing AI technologies. The global data annotation market is expected to grow significantly and continuously due to increased AI adoption by key industries.
According to LinkedIn, by 2025, 10% of IT spending is expected to focus on AI-related activities. The AI revolution relies on data annotation, and the global market for annotation tools is projected to reach USD 3.4 billion by 2028.
Automation tools will impact industries like healthcare, retail, autonomous vehicles, and finance in 2023.
Artificial Intelligence Annotation Is Here to Stay
Artificial intelligence (AI) is reshaping the IT industry and is here to stay. So, before delving into the world of AI, it’s important to understand fundamental processes, such as data annotation.
Data annotation, the practice of labeling data for machine learning, plays a pivotal role in training algorithms, providing essential input for discerning patterns.
Whether categorizing images for self-driving cars or labeling medical records for healthcare AI, data annotation is essential across various sectors.
The future of data annotation looks promising, with the global market projected to soar and automation paving the way for greater efficiency.
As AI continues to revolutionize industries, data annotation stands as a fundamental force driving the intelligence behind our evolving digital landscape. We hope this article provided you with some insights on AI data annotation!